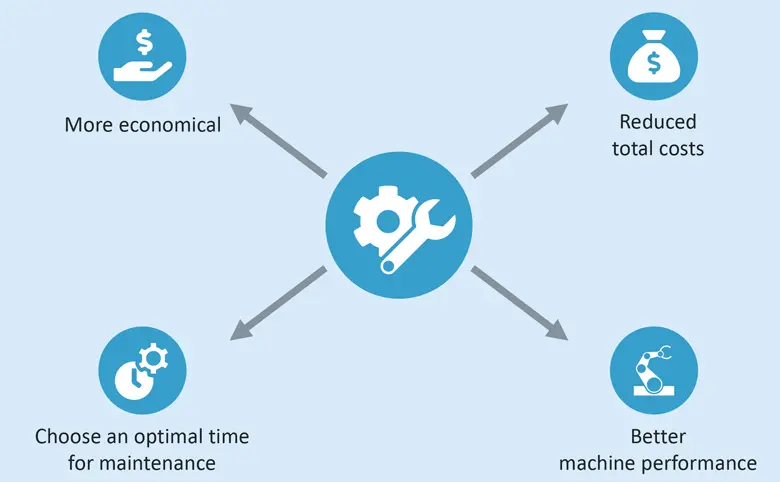
In the realm of Industrial Manufacturing, ensuring the reliability of equipment stands as a cornerstone for seamless operations. This article delves into the depths of Industrial Predictive Maintenance, a pivotal approach that enhances the dependability of machinery. Let’s embark on a journey to comprehend the significance and intricacies of this indispensable concept.
“Predictive maintenance keeps our machines healthy, ensuring uninterrupted production.”
Understanding Predictive Maintenance
Predictive Maintenance, in simple terms, is like having a smart companion for your machinery. Instead of waiting for a breakdown, it predicts potential issues. It’s akin to a health check-up for equipment, foreseeing problems before they become critical.
Why Predictive Maintenance Matters
- Minimizing Downtime Predictive Maintenance allows for timely intervention, reducing the downtime caused by unexpected breakdowns. Think of it as fixing a leak before it turns into a flood.
- Cost Efficiency Addressing issues proactively is not only about time but also about money. By fixing smaller problems early on, you prevent them from snowballing into costly repairs.
- Prolonging Equipment Life Just like regular exercise promotes a healthy life, predictive maintenance extends the lifespan of machinery by addressing issues before they lead to irreversible damage.
Technological Facets of Predictive Maintenance
Now, let’s peek into the technological wizardry that makes Predictive Maintenance a reality in the industrial landscape.
Sensors and IoT Integration
- Smart Sensors: The Watchful Eyes Smart sensors act as the eyes and ears of machinery, continuously monitoring parameters such as temperature, vibration, and performance metrics. These sensors provide real-time data, forming the basis for predictive analytics.
- IoT: Connecting the Dots The Internet of Things (IoT) takes the data from these sensors and turns it into actionable insights. It’s like a virtual brain that processes information and helps make informed decisions regarding maintenance needs.
Big Data Analytics
- Decoding Patterns Big Data Analytics crunches vast amounts of data to identify patterns and trends. It’s like finding a needle in a haystack, but with algorithms doing the heavy lifting.
- Predictive Algorithms: Crystal Balls of Maintenance Predictive algorithms use historical data to forecast potential issues. It’s like predicting the weather, but instead, we’re foreseeing the operational climate of machinery.
Implementation in Industrial Manufacturing
Integration Challenges
- Legacy Systems: Bridging the Gap Many industrial setups still operate on legacy systems. Integrating predictive maintenance requires a careful transition, ensuring the new complements the old seamlessly.
- Skill Set Upgrade: Nurturing Expertise Implementing predictive maintenance necessitates upskilling the workforce. It’s akin to providing a toolkit for a new job – the workforce needs the right skills to leverage this advanced approach.
Real-World Success Stories
- Aerospace Industry: Soaring High with Predictive Maintenance The aerospace sector utilizes predictive maintenance to ensure the safety and reliability of aircraft. Regular checks and timely interventions based on predictive insights contribute to accident prevention.
- Automotive Manufacturing: Driving Efficiency In the automotive industry, predictive maintenance minimizes production halts by addressing equipment issues before they escalate. This approach optimizes efficiency on the assembly line.
Key Strategies for Successful Implementation
Investing Wisely
Strategic Investment: The Backbone of Predicative Maintenance
The Backbone of Predictive Maintenance Investing in the right technology is crucial. It’s akin to choosing the right tools for a job – the better the investment, the smoother the process.
Continuous Monitoring: A Proactive Approach Predictive maintenance
Predictive maintenance is not a one-time effort; it’s an ongoing process. Continuous monitoring ensures that potential issues are addressed promptly, maintaining the overall health of the equipment.
Practical Application:
Imagine a factory that produces widgets using various machines. With Industrial Predictive Maintenance, the sensors on these machines continuously monitor their health. If a sensor detects an anomaly, let’s say unusual vibrations in a critical component, the system alerts the maintenance team. They can then schedule a preventive maintenance check before the issue worsens. This proactive approach avoids unexpected breakdowns, ensuring a smooth production process and minimizing downtime. In this way, Industrial Predictive Maintenance acts like a vigilant guardian, protecting the efficiency of the entire manufacturing operation.
Real-world Impact:
Consider a scenario in a power plant that relies on turbines for electricity generation. Implementing Industrial Predictive Maintenance significantly impacts the plant’s performance. By predicting potential turbine failures based on data from sensors, the plant can schedule maintenance during low-demand periods. This prevents unplanned shutdowns, ensuring a continuous and reliable power supply to the community. The real-world impact is evident – fewer power outages, increased reliability, and a more efficient energy infrastructure.
“In the industrial landscape, foresight is power – that’s what predictive maintenance provides.”
Conclusion: A Future of Reliability
In conclusion, Industrial Predictive Maintenance emerges as the linchpin for enhancing equipment reliability. From the integration of sensors and IoT to the intricate dance of big data analytics, this approach revolutionizes how industries ensure the longevity of their machinery. As we navigate the ever-evolving landscape of Industrial Manufacturing, predictive maintenance stands as a beacon, guiding us towards a future where equipment breakdowns are mere echoes of the past. Embrace the power of foresight – invest in Predictive Maintenance for a robust and dependable industrial ecosystem.